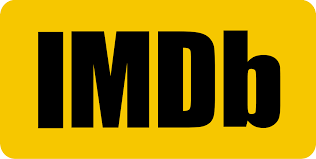
Designing the relational database
In this series of blog posts we will present an end-to-end database project using MySQL the IMDb dataset. This is the second in the series of posts on my database project. In this post we will present the Entity-Relationship (ER) and logical schema diagrams for our relational database. We will also discuss the Extract-Transform-Load (ETL) tasks we performed using python. Today we will not go into the details of how to design a normalised database as this can take up a large portion of a university level course on databases. Instead we will refer the interested reader to the fantastic book by Ramakrishnan and Gehrke [1] and the incredibly useful set of video lectures for the course CMPSC431W: Database Management Systems taught at Penn State by Yu-San Lin [2]. We will now present our design.
For this project we used yEd to create our Entity-Relationship (ER) and logical schema diagrams.
“yEd is a powerful desktop application that can be used to quickly and effectively generate high-quality diagrams. Create diagrams manually, or import your external data for analysis. Our automatic layout algorithms arrange even large data sets with just the press of a button.”
“yEd is freely available and runs on all major platforms: Windows, Unix/Linux, and macOS.”
We really liked this software. It was very easy to use with simple GUI.
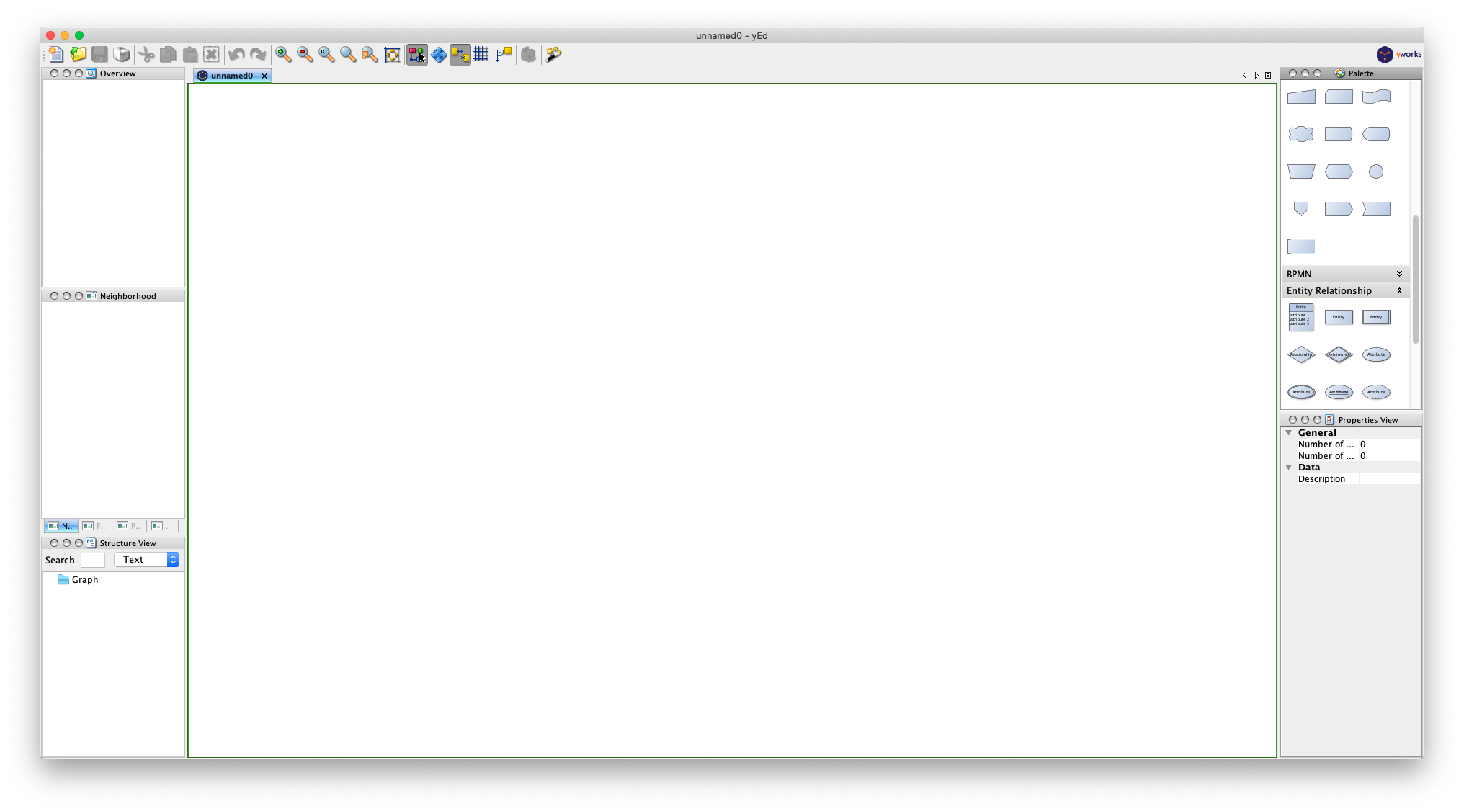
Entity-Relationship (ER) diagram
The IMDb data as provided is not normalised. We designed the entity-relationship diagram for our IMDb relational database. This was created using yEd and is shown below.
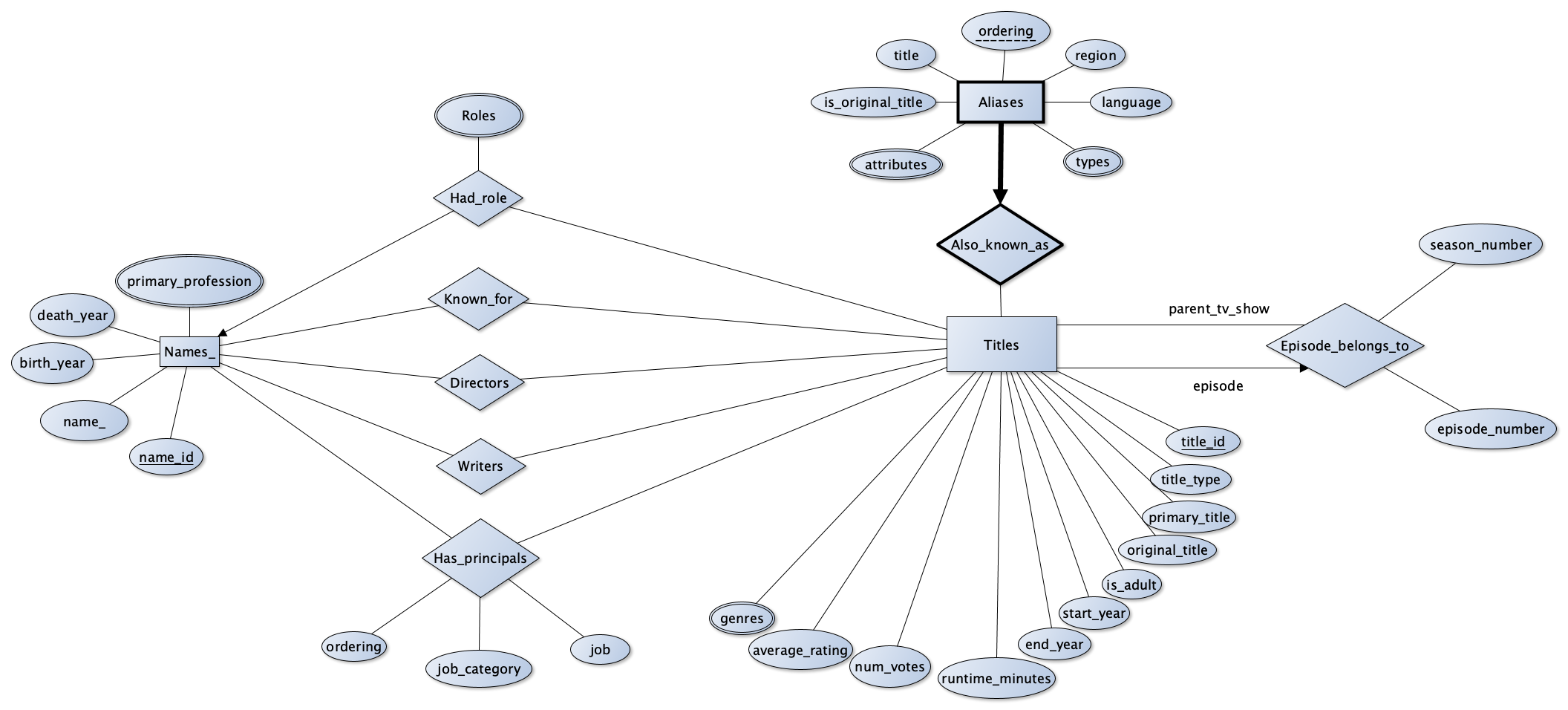
As one can see it is a reasonably complex database, but, not as complex as one may find in production business systems. However, it will suffice for our purposes.
Logical schema
We then normalise our ER diagram and obtain the logical schema illustrated below. Note the following:
-
New tables were created for multi-valued attributes, such as Title_genres.
-
We pulled the rating information attributes from the Titles entity, because many titles didn’t have a rating. If we were to store them in the Titles table, then we would have stored many NULL values. Instead we decided to separate this information, by putting it into the table Title_ratings.
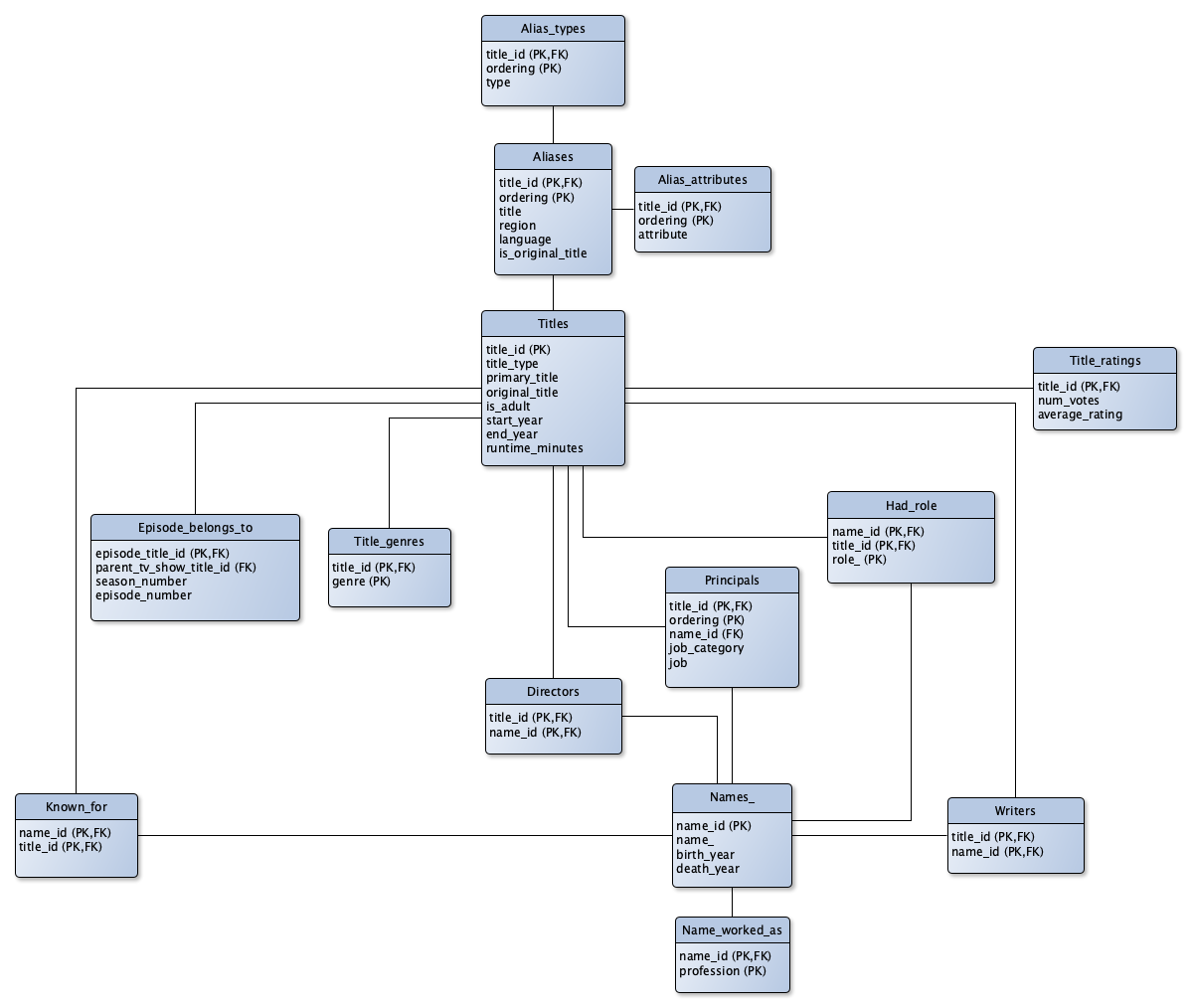
This diagram was also created using yEd. Note that in this diagram we have denote primary keys by PK and foreign keys by FK.
Extract-Transform-Load: Preparing the IMDb data
The ETL part of this project was done using a single python script. The script imdb_converter.py
reads in the 7 data files, cleans and normalises the IMDb data. After which, the
desired set of tables are output as tab-separate-value (tsv) files. It was written
to be as modular as possible with separate functions written to perform specific
tasks. The functions that were implemented are:
- unzip_files(folder)
- make_Aliases(title_akas)
- make_Alias_types(title_akas)
- make_Alias_attributes(title_akas)
- make_Directors_and_Writers(title_crew)
- make_Episode_belongs_to(title_episode)
- make_Names_(name_basics)
- make_Name_worked_as(name_basics)
- make_Known_for(name_basics)
- make_Principals(title_principals)
- make_Had_role(title_principals)
- make_Titles(title_basics)
- make_Title_genres(title_basics)
- make_Title_ratings(title_ratings)
From the names and the comments their purpose should be clear. The python script is show below
# Imports
# -------
import numpy as np
import pandas as pd
import os
import gzip
import shutil
# Helper functions
#------------------
# Used to unzip IMDb data files
def unzip_files(folder):
"""
unzip_files():
==============
Unzip all gzipped files in folder.
INPUT:
======
folder (string) - Path to folder containing the zip files.
OUTPUT:
=======
out_files (array of strings) - Paths to unzipped files.
"""
out_files = []
# For each file in folder
for file in os.listdir(folder):
# If it is gzipped unzip it
if file.endswith(".gz"):
in_file_path = os.path.join(folder, file)
out_file_path = os.path.join(folder,file.replace('.gz',''))
out_files.append(out_file_path)
print('\tUnzipping ',in_file_path,' to ',out_file_path)
# Unzip current file
with gzip.open(in_file_path, 'rb') as f_in:
with open(out_file_path, 'wb') as f_out:
shutil.copyfileobj(f_in, f_out)
return out_files
#-------------------------------------------------------------------------------
# Functions to process IMDb data
#-------------------------------
# Create Aliases table
def make_Aliases(title_akas):
"""
make_Aliases():
===============
Creates Aliases table from from title.akas.tsv.
INPUT:
======
title_akas (pandas dataframe) - Contains the title.akas.tsv data table.
"""
# title.akas.tsv
# FORMAT: ['titleId', 'ordering', 'title', 'region', 'language', 'types',
# 'attributes', 'isOriginalTitle']
print("\tMaking 'Aliases' table")
# Extract columns
Aliases = title_akas[['titleId','ordering','title','region','language',
'isOriginalTitle']]
# Rename columns
Aliases = Aliases.rename(columns={
'titleId':'title_id',
'isOriginalTitle':'is_original_title'
})
# Output to file
Aliases.to_csv('Aliases.tsv',index=False,na_rep=r'\N',sep='\t')
#-------------------------------------------------------------------------------
# Create Alias_types table
def make_Alias_types(title_akas):
"""
make_Alias_types():
===================
Creates Alias_types table from from title.akas.tsv.
INPUT:
======
title_akas (pandas dataframe) - Contains the title.akas.tsv data table.
"""
# title.akas.tsv
# FORMAT: ['titleId', 'ordering', 'title', 'region', 'language', 'types',
# 'attributes', 'isOriginalTitle']
print("\tMaking 'Alias_types' table")
# Extract columns
Alias_types = title_akas[['titleId','ordering','types']]
# Rename columns
Alias_types = Alias_types.rename(columns={
'titleId':'title_id',
'types':'type'
})
# types is said to be an array. In the data we have this appears to not be
# true. There appears to be only one string for each pair of titleId and
# ordering values. There are many NULL (\N) values (~95%) in this field. We
# don't keep these NULL values. Only entries with non-NULL values in the
# field are kept and if if there is no entry in this table for a given
# title_id and ordering pair it is considered to be NULL.
Alias_types = Alias_types.dropna()
# Output to file
Alias_types.to_csv('Alias_types.tsv',index=False,na_rep=r'\N',sep='\t')
#-------------------------------------------------------------------------------
# Create Alias_attributes table
def make_Alias_attributes(title_akas):
"""
make_Alias_attributes():
========================
Creates Alias_attributes table from from title.akas.tsv.
INPUT:
======
title_akas (pandas dataframe) - Contains the title.akas.tsv data table.
"""
# title.akas.tsv
# FORMAT: ['titleId', 'ordering', 'title', 'region', 'language', 'types',
# 'attributes', 'isOriginalTitle']
print("\tMaking 'Alias_attributes' table")
# Extract columns
Alias_attributes = title_akas[['titleId','ordering','attributes']]
# Rename columns
Alias_attributes = Alias_attributes.rename(columns={
'titleId':'title_id',
'attributes':'attribute'
})
# attributes is said to be an array. In the data we have this appears to not
# be true. There appears to be only one string for each pair of titleId and
# ordering values. There are many NULL (\N) values (~99%) in this field. We
# don't keep these NULL values. Only entries with non-NULL values in the
# field are kept and if if there is no entry in this table for a given
# title_id and ordering pair it is considered to be NULL.
Alias_attributes = Alias_attributes.dropna()
# Output to file
Alias_attributes.to_csv('Alias_attributes.tsv',index=False,na_rep=r'\N',sep='\t')
#-------------------------------------------------------------------------------
# Create Directors and Writers tables
def make_Directors_and_Writers(title_crew):
"""
make_Directors_and_Writers():
=============================
Creates the Directors and Writers tables from title.crew.tsv.
INPUT:
======
title_crew (pandas dataframe) - Contains the title.crew.tsv data table.
"""
# title.crew.tsv
# FORMAT: ['tconst', 'directors', 'writers']
print("\tMaking 'Directors' and 'Writers' tables")
# Here we have directors and writers which contain a comma separated list of
# nconst. We will output title_id (tconst), name_id (nconst)
Directors = title_crew[['tconst','directors']]
Writers = title_crew[['tconst','writers']]
# Rename columns
Directors = Directors.rename(columns={
'tconst':'title_id',
'directors':'name_id'
})
Writers = Writers.rename(columns={
'tconst':'title_id',
'writers':'name_id'
})
# Drop NULL entries
Directors = Directors.dropna()
Writers = Writers.dropna()
# Explode name_id into separate entries
Directors = Directors.assign(name_id=Directors.name_id.str.split(',')).explode('name_id').reset_index(drop=True)
Writers = Writers.assign(name_id=Writers.name_id.str.split(',')).explode('name_id').reset_index(drop=True)
# Output to file
Directors.to_csv('Directors.tsv',index=False,na_rep=r'\N',sep='\t')
Writers.to_csv('Writers.tsv',index=False,na_rep=r'\N',sep='\t')
#-------------------------------------------------------------------------------
# Create Episode_belongs_to table
def make_Episode_belongs_to(title_episode):
"""
make_Episode_belongs_to():
==========================
Creates the Episode_belongs_to table from title.episode.tsv.
INPUT:
======
title_episode (pandas dataframe) - Contains the title.episode.tsv data table.
"""
# title.episode.tsv
# FORMAT: ['tconst', 'parentTconst', 'seasonNumber', 'episodeNumber']
print("\tMaking 'Episode_belongs_to' table")
# No change other than column names
# Rename columns
Episode_belongs_to = title_episode.rename(columns={
'tconst':'title_id',
'parentTconst':'parent_tv_show_title_id',
'seasonNumber':'season_number',
'episodeNumber':'episode_number'
})
# Output to file
Episode_belongs_to.to_csv('Episode_belongs_to.tsv',index=False,na_rep=r'\N',sep='\t')
#-------------------------------------------------------------------------------
# Create Names_ table
def make_Names_(name_basics):
"""
make_Names_():
==============
Creates the Names_ table from name.basics.tsv.
INPUT:
======
name_basics (pandas dataframe) - Contains the name.basics.tsv data table.
"""
# name.basics.tsv has columns:
# FORMAT: ['nconst', 'primaryName', 'birthYear', 'deathYear',
# 'primaryProfession', 'knownForTitles']
print("\tMaking 'Names_' table")
# Extract columns from name_basics for Names_ table
Names_ = name_basics[['nconst','primaryName','birthYear','deathYear']]
# Rename columns
Names_= Names_.rename(columns={
'nconst':'name_id',
'primaryName':'name_',
'birthYear':'birth_year',
'deathYear':'death_year'
})
# Output to file
Names_.to_csv('Names_.tsv',index=False,na_rep=r'\N',sep='\t')
#-------------------------------------------------------------------------------
# Create Name_worked_as table
def make_Name_worked_as(name_basics):
"""
make_Name_worked_as():
======================
Creates the Name_worked_as table from name.basics.tsv.
INPUT:
======
name_basics (pandas dataframe) - Contains the name.basics.tsv data table.
"""
# name.basics.tsv has columns:
# FORMAT: ['nconst', 'primaryName', 'birthYear', 'deathYear',
# 'primaryProfession', 'knownForTitles']
print("\tMaking 'Name_worked_as' table")
# Extract columns from name_basics for Name_worked_as table
Name_worked_as = name_basics[['nconst','primaryProfession']]
# There are NaN values, drop them? Yes, because both are used as a primary key
Name_worked_as = Name_worked_as.dropna()
# Rename columns
Name_worked_as = Name_worked_as.rename(columns={
'nconst':'name_id',
'primaryProfession':'profession'
})
# primaryProfessions is a comma separated string, we need to 'explode' this
Name_worked_as = Name_worked_as.assign(profession=Name_worked_as.profession.str.split(',')).explode('profession').reset_index(drop=True)
# Output to file
Name_worked_as.to_csv('Name_worked_as.tsv',index=False,na_rep=r'\N',sep='\t')
#-------------------------------------------------------------------------------
# Create Known_for table
def make_Known_for(name_basics):
"""
make_Known_for():
=================
Creates Known_for table from name.basics.tsv.
INPUT:
======
name_basics (pandas dataframe) - Contains the name.basics.tsv data table.
"""
# name.basics.tsv has columns:
# FORMAT: ['nconst', 'primaryName', 'birthYear', 'deathYear',
# 'primaryProfession', 'knownForTitles']
print("\tMaking 'Known_for' table")
# Extract columns from name_basics for Known_for table
Known_for = name_basics[['nconst','knownForTitles']]
# There are NaN values, drop them? Yes, because both are used as a primary key
Known_for = Known_for.dropna()
# Rename columns
Known_for = Known_for.rename(columns={
'nconst':'name_id',
'knownForTitles':'title_id'
})
# knownForTitles is a comma separated string, we need to 'explode' this
Known_for = Known_for.assign(title_id=Known_for.title_id.str.split(',')).explode('title_id').reset_index(drop=True)
# Output to file
Known_for.to_csv('Known_for.tsv',index=False,na_rep=r'\N',sep='\t')
#-------------------------------------------------------------------------------
# Create Principals table
def make_Principals(title_principals):
"""
make_Principals():
==================
Create Principals table from title.principals.tsv.
INPUT:
======
title_principals (pandas dataframe) - Contains the title.principals.tsv data table.
"""
# title.principals.tsv
# FORMAT: ['tconst', 'ordering', 'nconst', 'category', 'job', 'characters']
print("\tMaking 'Principals' table")
# Extract columnns
Principals = title_principals[['tconst','ordering','nconst','category','job']]
# Rename columns
Principals = Principals.rename(columns={
'tconst':'title_id',
'nconst':'name_id',
'category':'job_category',
})
# Output to file
Principals.to_csv('Principals.tsv',index=False,na_rep=r'\N',sep='\t')
#-------------------------------------------------------------------------------
# Create Had_role table
def make_Had_role(title_principals):
"""
make_Had_role():
================
Create Had_role table from title.principals.tsv.
INPUT:
======
title_principals (pandas dataframe) - Contains the title.principals.tsv data table.
"""
# title.principals.tsv
# FORMAT: ['tconst', 'ordering', 'nconst', 'category', 'job', 'characters']
print("\tMaking 'Had_role' table")
# Extract columns
Had_role = title_principals[['tconst','nconst','characters']]
# Rename columns
Had_role = Had_role.rename(columns={
'tconst':'title_id',
'nconst':'name_id',
'characters':'role_'
})
# Drop NULL entries (~50%)
Had_role = Had_role.dropna()
# role_ entries are formatted as ["Mum","Tidy Ted","Fang"], we will remove
# all "[]"
Had_role['role_'] = Had_role['role_'].str.replace('[\"\[\]]','',regex=True)
# also replace slashes \ with vertical bars |
Had_role['role_'] = Had_role['role_'].str.replace('\\','|')
# and explode the list into separate entries
Had_role = Had_role.assign(role_=Had_role.role_.str.split(',')).explode('role_').reset_index(drop=True)
# There is some duplicate data, this is easily seen
#Had_role[Had_role.duplicated()]
# See a specific example
#Had_role[Had_role['title_id'].str.contains('tt0003960') & Had_role['name_id'].str.contains('nm0220672') & Had_role['role_'].str.contains('Ballerina')]
# Slight character font differences which lead to more duplicates. Not
# considered a duplicate in python, but MySQL cannot tell the difference
# unless you change the character set! We could do the following
#Had_role['role_'] = Had_role['role_'].str.replace('ì','i')
#Had_role['role_'] = Had_role['role_'].str.replace('ä','a')
# however, we do not bother to remove these or others.
# Also, MySQL cannot tell the difference between lower and upper case unless
# you change the character set! There are some entries which differ only by
# capitalisation, so we ensure that every entry has title capitalisation.
Had_role['role_'] = Had_role['role_'].str.title()
# Remove spaces at the start and end of an value
Had_role['role_'] = Had_role['role_'].str.replace('^ | $','',regex=True)
# Drop these duplicates
Had_role.drop_duplicates(keep='first',inplace=True)
# Output to file
Had_role.to_csv('Had_role.tsv',index=False,na_rep=r'\N',sep='\t')
#-------------------------------------------------------------------------------
# Create Titles table
def make_Titles(title_basics):
"""
make_Titles():
==============
Create Titles table from title.basics.tsv.
INPUT:
======
title_basics (pandas dataframe) - Contains the title.basics.tsv data table.
"""
# title.basics.tsv
# FORMAT: ['tconst', 'titleType', 'primaryTitle', 'originalTitle',
# 'isAdult', 'startYear', 'endYear', 'runtimeMinutes', 'genres']
print("\tMaking 'Titles' table")
# Extract columns from title_basics for Titles table
Titles = title_basics[['tconst','titleType','primaryTitle',
'originalTitle', 'isAdult', 'startYear', 'endYear', 'runtimeMinutes']]
# Rename columns
Titles = Titles.rename(columns={
'tconst':'title_id',
'titleType':'title_type',
'primaryTitle':'primary_title',
'originalTitle':'original_title',
'isAdult':'is_adult',
'startYear':'start_year',
'endYear':'end_year',
'runtimeMinutes':'runtime_minutes'
})
# Output to file
Titles.to_csv('Titles.tsv',index=False,na_rep=r'\N',sep='\t')
#-------------------------------------------------------------------------------
# Create Title_genres table
def make_Title_genres(title_basics):
"""
make_Title_genres():
====================
Create Title_genres table from title.basics.tsv.
INPUT:
======
title_basics (pandas dataframe) - Contains the title.basics.tsv data table.
"""
# title.basics.tsv
# FORMAT: ['tconst', 'titleType', 'primaryTitle', 'originalTitle',
# 'isAdult', 'startYear', 'endYear', 'runtimeMinutes', 'genres']
print("\tMaking 'Title_genres' table")
# Extract columns
Title_genres = title_basics[['tconst','genres']]
# Rename columns
Title_genres = Title_genres.rename(columns={
'tconst':'title_id',
'genres':'genre'
})
# There are NaN values, drop them? Yes, because both are used as a primary key
Title_genres = Title_genres.dropna()
# genres is a comma separated string, we need to 'explode' this
Title_genres = Title_genres.assign(genre=Title_genres.genre.str.split(',')).explode('genre').reset_index(drop=True)
# Output to file
Title_genres.to_csv('Title_genres.tsv',index=False,na_rep=r'\N',sep='\t')
#-------------------------------------------------------------------------------
# Create Title_ratings (just renaming columns)
def make_Title_ratings(title_ratings):
"""
make_Title_ratings():
=====================
Create Title_ratings (just renaming columns) from title.ratings.tsv.
INPUT:
======
title_ratings (pandas dataframe) - Contains the title.ratings.tsv data table.
"""
# title.ratings.tsv
# FORMAT: ['tconst', 'averageRating', 'numVotes']
print("\tMaking 'Title_ratings' table")
# Rename columns
Title_ratings = title_ratings.rename(columns={
'tconst':'title_id',
'averageRating':'average_rating',
'numVotes':'num_votes'
})
# Output to file
Title_ratings.to_csv('Title_ratings.tsv',index=False,na_rep=r'\N',sep='\t')
#------------------------ END OF FUNCTION DEFINITIONS --------------------------
# Set path to IMDb data
# ---------------------
data_path = './imdb_data'
print('Looking for IMDb data in: ',data_path,'\n')
# Unzip IMDb data files
#----------------------
data_files = unzip_files(data_path)
# Read in and process all IMDb data files
# ---------------------------------------
# title.akas.tsv
#----------------
# FORMAT: ['titleId', 'ordering', 'title', 'region', 'language', 'types',
# 'attributes', 'isOriginalTitle']
# Read title.akas
print('\n','Reading title.akas.tsv ...','\n')
title_akas = pd.read_csv(os.path.join(data_path,'title.akas.tsv'),
dtype = {'titleId':'str', 'ordering':'int', 'title':'str', 'region':'str',
'language':'str', 'types':'str','attributes':'str',
'isOriginalTitle':'Int64'},
sep='\t',na_values='\\N',quoting=3)
# Make tables
make_Aliases(title_akas)
make_Alias_types(title_akas)
make_Alias_attributes(title_akas)
# Delete title_akas, no longer need this dataframe
del title_akas
# title.crew.tsv
#----------------
# FORMAT: ['tconst', 'directors', 'writers']
print('\n','Reading title.crew.tsv','\n')
# Read title.crew
title_crew = pd.read_csv(os.path.join(data_path,'title.crew.tsv'),sep='\t',na_values='\\N')
# Make table
make_Directors_and_Writers(title_crew)
# Delete title_crew, no longer need this dataframe
del title_crew
# title.episode.tsv
#------------------
# FORMAT: ['tconst', 'parentTconst', 'seasonNumber', 'episodeNumber']
print('\n','Reading title.episode.tsv ...','\n')
# Read title.episode
title_episode = pd.read_csv(os.path.join(data_path,'title.episode.tsv'),
dtype = {'tconst':'str', 'parentTconst':'str', 'seasonNumber':'Int64',
'episodeNumber':'Int64'},
sep='\t',na_values='\\N')
# Make table
make_Episode_belongs_to(title_episode)
# Delete title_episode, no longer need this dataframe
del title_episode
# name.basics.tsv
#-----------------
# FORMAT: ['nconst', 'primaryName', 'birthYear', 'deathYear',
# 'primaryProfession', 'knownForTitles']
print('\n','Reading name.basics.tsv ...','\n')
# Read name.basics
name_basics = pd.read_csv(os.path.join(data_path,'name.basics.tsv'),
dtype = {'nconst':'str', 'primaryName':'str', 'birthYear':'Int64',
'deathYear':'Int64', 'primaryProfession':'str', 'knownForTitles':'str'},
sep='\t',na_values='\\N')
# Make tables
make_Names_(name_basics)
make_Name_worked_as(name_basics)
make_Known_for(name_basics)
# Delete name_basics, no longer need this dataframe
del name_basics
# title.principals.tsv
#---------------------
# FORMAT: ['tconst', 'ordering', 'nconst', 'category', 'job', 'characters']
print('\n','Reading title.principals.tsv ...','\n')
# Read title.principals
title_principals = pd.read_csv(os.path.join(data_path,'title.principals.tsv'),sep='\t',na_values='\\N')
# Make tables
make_Principals(title_principals)
make_Had_role(title_principals)
# Delete title_principals, no longer need this dataframe
del title_principals
# title.basics.tsv
#------------------
# FORMAT: ['tconst', 'titleType', 'primaryTitle', 'originalTitle', 'isAdult',
# 'startYear', 'endYear', 'runtimeMinutes', 'genres']
# quoting = 3 used to ignore quotation marks, done because in some cases the was
# one missing, e.g., primaryTitle was "Rolling in the Deep Dish
print('\n','Reading title.basics.tsv ...','\n')
# Read title.basics
title_basics = pd.read_csv(os.path.join(data_path,'title.basics.tsv'),
dtype = {'tconst':'str', 'titleType':'str', 'primaryTitle':'str',
'originalTitle':'str', 'isAdult':'int', 'startYear':'Int64',
'endYear':'Int64', 'runtimeMinutes':'Int64', 'genres':'str'},
sep='\t',na_values='\\N',quoting=3)
# Make tables
make_Titles(title_basics)
make_Title_genres(title_basics)
# Delete title_basics, no longer need this dataframe
del title_basics
# title.ratings.tsv
#-------------------
# FORMAT: ['tconst', 'averageRating', 'numVotes']
print('\n','Reading title.ratings.tsv ...','\n')
# Read title.ratings
title_ratings = pd.read_csv(os.path.join(data_path,'title.ratings.tsv'),sep='\t',na_values='\\N')
# Make table
make_Title_ratings(title_ratings)
# Delete title_ratings, no longer need this dataframe
del title_ratings
The python script was written to be as modular as possible and made use of the pandas and numpy libraries. Running this python script in the terminal we get the following output:
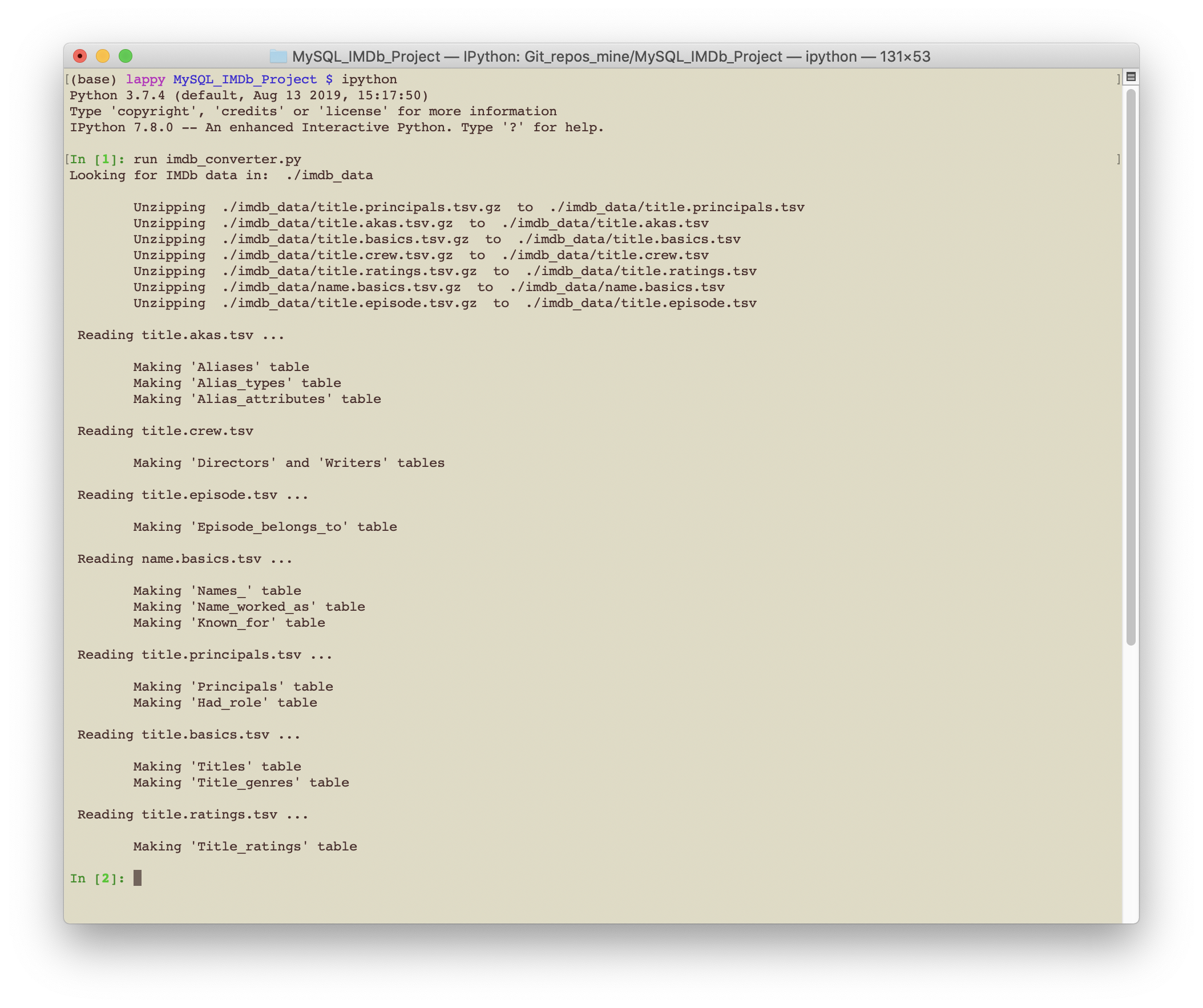
Conclusion
In this post we designed a relational database to store the IMDb dataset, used yEd to create the ER and logical schema diagrams and used python to perform ETL tasks. In the next post, the third in the series, we will create the database in MySQL we designed using SQL scripts, load the data into the database, add primary and foreign key constraints and index the database. The code and images for this project are shared in the GitHub repository.
Further Reading
-
R. Ramakrishnan and J. Gehrke, Database management systems, 3rd edition, Mc Graw-Hill. Companion site
-
Yu-San Lin, CMPSC431W: Database Management Systems fall 2015 video lectures, Penn State. Course site